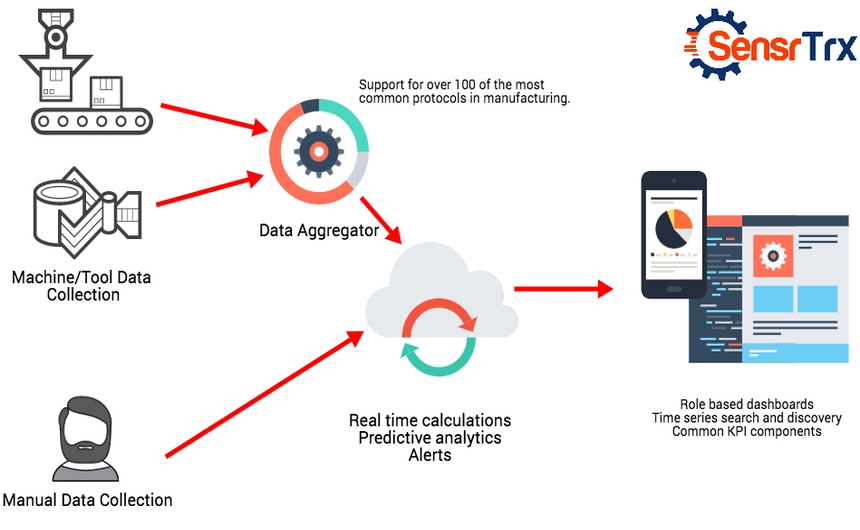
According to Dheeraj Remella, the value of big data analytics lies in the ability to embed the insights gained from data models into other applications to support decision-making. To achieve this, it is crucial to present insights in a format that can be easily incorporated into real-time decision-making processes, allowing for in-the-moment decisions based on streaming event data.
Data wrangling, which involves cleaning, labeling, and organizing data for analytics, requires seamless integration across different data sources, applications, and APIs. Big data analytics tools must support various data types, protocols, and integration scenarios to simplify and accelerate the data wrangling process while ensuring secure access to the data based on roles and permissions.
In the data analytics process, exploration plays a vital role in understanding the business context and formulating better analytic questions. Streamlining this exploration phase with helpful features enables organizations to test new hypotheses faster and discover valuable connections hidden within the data.
Supporting different types of analytics, such as business intelligence, predictive analytics, real-time analytics, and machine learning, is essential for a well-rounded big data analytics tool. Such flexibility allows businesses to leverage diverse use cases with minimal effort and without the need for extensive retraining when adopting different tools.
Scalability is a key requirement for big data analytics tools, as they must handle large data sets and high data ingestion rates without incurring exorbitant hardware or cloud service costs. The ability to scale algorithms effortlessly from small to large data sets is also critical for efficient data analysis.
Version control is crucial in large data analytics projects to track changes made by multiple individuals to the model parameters. Having version control capabilities ensures easy rollback to a previous working version if unexpected problems arise during the production phase.
Simple integration capabilities in big data analytics tools facilitate easy sharing of results with other developers and data scientists. These tools should seamlessly integrate with existing enterprise and cloud applications and data warehouses.
A robust data management platform is essential for maintaining consistency and standardization across all deliverables in big data analytics. It helps enterprises maintain a single source of truth, a critical aspect of successful data initiatives.
Data governance features are vital to ensure compliance and security in big data analytics tools. These features help track the source and characteristics of data sets used in building analytic models and manage data used by data scientists and engineers. Data governance is particularly crucial for handling sensitive data subject to privacy regulations.
Data processing frameworks that support both analytics and data processing are advantageous for developers and data scientists. These frameworks enable real-time processing, complex extract-transform-load tasks, machine learning, reporting, and SQL operations using the same set of tools, facilitating the highly iterative data science process.
In conclusion, a comprehensive big data analytics tool should encompass the above features to provide valuable insights, support real-time decision-making, and enable seamless data exploration and integration across diverse use cases with scalability, version control, data management, and data governance functionalities.